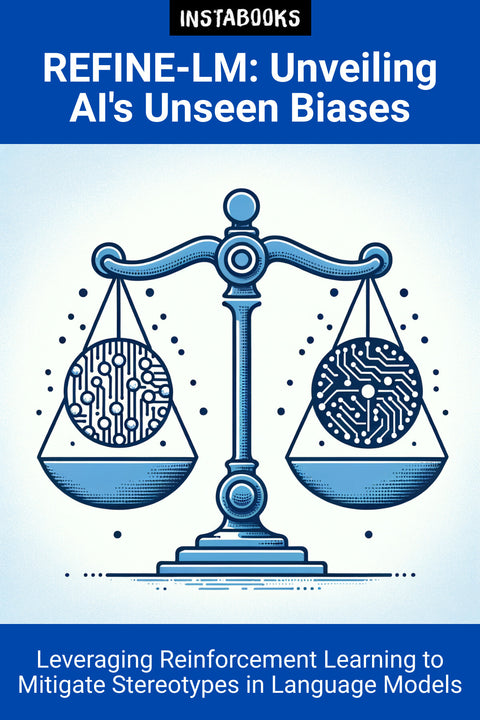
REFINE-LM: Unveiling AI's Unseen Biases
Premium AI Book - 200+ pages



Understanding the Landscape of Bias in Language Models
In today's rapidly evolving world of artificial intelligence, Large Language Models (LLMs) such as BERT and GPT are paving the way for new technological advancements. However, an undercurrent of bias often accompanies these LLMs, manifesting in various forms such as gender, geographical, and racial biases. Addressing these biases is crucial for ensuring ethical and fair AI applications, and that's where this book comes in.
Exploring Existing Solutions and Their Limitations
Previous attempts to tackle bias in LLMs have largely revolved around data preprocessing and embedding debiasing. While these methods offer some relief, they are often resource-heavy and not sufficiently versatile across different biases. This section dives into these existing solutions, examining their successes and constraints, and paving the path for a novel approach through REFINE-LM.
Introducing REFINE-LM: A Paradigm Shift in Debiasing
At the heart of this book is a thorough examination of REFINE-LM, a groundbreaking post-hoc filtering method. By utilizing reinforcement learning, REFINE-LM offers a bias-type agnostic solution that doesn’t necessitate model fine-tuning or extensive human annotations. Learn about the methodology, how it operates above the LLM's word probability distribution, and why it represents a substantial advancement in mitigating stereotypes without demanding significant computational resources.
Evaluating the Performance and Efficacy of REFINE-LM
Drawing on extensive experiments across multiple models like DistillBERT, BERT, and RoBERTa, this book showcases how REFINE-LM not only diminishes stereotypes but also maintains the integrity of language models' performance. Understand how this method outperforms others in terms of bias reduction across contexts such as gender, ethnicity, and nationality, all while requiring fewer training resources.
Navigating Limitations and Ethical Considerations
Finally, no solution is without challenges. Discover the current limitations of REFINE-LM, such as its need for individualized adaptations for diverse architectures and its ability to handle one bias type per implementation. Moreover, delve into the ethical considerations necessary when deploying REFINE-LM, ensuring its use aligns with ethical norms and addresses the specific biases relevant to individual contexts.
Table of Contents
1. The Origin and Impact of Bias in LLMs- Understanding Bias Formation
- Historical Examples of AI Bias
- The Societal Impact of Biased LLMs
2. Traditional Debiasing Techniques
- Data Preprocessing Methods
- Embedding Debiasing Strategies
- Limitations and Challenges
3. Introducing REFINE-LM
- Conceptual Framework
- Core Principles of REFINE-LM
- Initial Development and Testing
4. REFINE-LM Methodology in Detail
- Reinforcement Learning Basics
- Constructing the Debiasing Model
- Integration with Pre-existing LLMs
5. Performance Benefits of REFINE-LM
- Bias Reduction Achievements
- Comparative Performance Analysis
- Case Studies Across Models
6. Resource Efficiency in Debiasing
- Comparison with Traditional Methods
- Computational Resource Savings
- Practical Implementations
7. Limitations of REFINE-LM
- Single Bias Type Handling
- Complex Integration Needs
- Stability and Scalability Issues
8. Ethical Considerations in Debiasing
- Defining Acceptable Bias Levels
- Real-world Implications
- Ongoing Ethical Debates
9. Adaptive Strategies for Different Biases
- Refining for Auto-regressive Models
- Layer Stacking Techniques
- Customized Solutions
10. Future Prospects and Research Directions
- Innovative Debiasing Approaches
- Frontiers in Reinforcement Learning
- Speculative Applications in AI
11. Practical Applications and Use Cases
- Industry-Specific Implementations
- Academic Research Contributions
- Collaborative Initiatives
12. Conclusion and Reflections
- Summarizing Key Insights
- Reflections on Ethical AI
- Future Visions for Bias-Free LLMs
Target Audience
AI ethics researchers, data scientists, NLP practitioners, and technology policymakers interested in AI bias mitigation.
Key Takeaways
- Understand the causes and impacts of bias in language models.
- Explore the limitations of traditional debiasing techniques.
- Learn about REFINE-LM, a reinforcement learning-based approach to debiasing.
- Evaluate REFINE-LM's performance and resource efficiency.
- Navigate ethical considerations in deploying debiasing methods.
- Discover future research directions in AI bias mitigation.
How This Book Was Generated
This book is the result of our advanced AI text generator, meticulously crafted to deliver not just information but meaningful insights. By leveraging our AI story generator, cutting-edge models, and real-time research, we ensure each page reflects the most current and reliable knowledge. Our AI processes vast data with unmatched precision, producing over 200 pages of coherent, authoritative content. This isn’t just a collection of facts—it’s a thoughtfully crafted narrative, shaped by our technology, that engages the mind and resonates with the reader, offering a deep, trustworthy exploration of the subject.
Satisfaction Guaranteed: Try It Risk-Free
We invite you to try it out for yourself, backed by our no-questions-asked money-back guarantee. If you're not completely satisfied, we'll refund your purchase—no strings attached.